PI: Helen Armstrong.
This past year, I worked on a research project with Dr. Matthew Peterson and research assistants Ashley Anderson, Kayla Rondinelli, and Isha Parate. In collaboration with The Laboratory for Analytic Sciences, we explored interface design, generative A.I., memory, and sensemaking.
Building on UX/UI research with language analysts conducted the previous spring, we used text-to-image generative AI to create compact visual representations. These representations are meant to activate analyst memory traces in ways that extend human memory. We called these compact visual representations “conceptual pegs.”
Imagine that you are an intelligence analyst. As you view mounds of documents, you come across a bit of information that you find significant in some way. Perhaps, it is an anomaly that you can’t quite explain. Perhaps the data itself is interesting, but you don’t see how it fits any current storylines. Perhaps the data strikes a cord with you, but you are unsure why. To capture this information, you quickly create a conceptual peg—without interrupting your normal workflow. Or, perhaps, the A.I. suggests that a conceptual peg be generated for a particular bit of information that the A.I. deems significant.
These pegs are then collected to the side and clustered by the A.I. As an analyst, each time you later glance at a peg, your mind traces back to your initial encounter with the information. In addition, you can use the pegs to query information, seeking unusual data connections. The A.I. can even cluster accumulated pegs for you to suggest possible connections between disparate information.
This, in summary, is the “Insights” project. Our official project goal: “Reveal potential innovations in interface design that will increase the likelihood of intelligence analysts recognizing important data connections with the help of machine learning by exploring an unconventional usage of visual representations to activate analyst memory traces.”
Some of the questions we addressed:
- How might an analyst intuitively generate pegs as part of their current workflow?
- How might an AI suggest concepts of interest?
- To what degree might an analyst and/or AI have agency over concept selection and visualization?
- How the analyst might unpack pegs to reawaken memories of raw data?
- How might the analyst and/or AI suggest associations among pegs to contribute to sensemaking?
Research Outcomes:
We produced 30 distinct multi-screen user interface studies and in a discovery process abstracted out approximately eight embedded features and facets of each imagined UI, as well as a comparable number of insights that could inform other UIs.
These abstractions of function permitted the classification of UI studies in relation to one another, and the extraction of useful concepts, which we then clustered into sets of features that were compatible with one another. These feature sets became possible user interfaces that were more robust than the individual UIs from the exploratory studies.
Having characterized these possible UIs so deliberately, we were able to devise a set of three interface concepts that address, in distinct ways, many of the unresolved questions we had — and still have — about how an AI might generate and arrange conceptual pegs, and about how analysts might be more or less involved in aspects of concept selection and concept visualization.
For our final outcomes we generated three scenario videos to demonstrate these interfaces concepts in use. (see videos below).
In addition to the research and scenario videos shared below, our work produced the Pictorial Trapezoid. This trapezoid provides a framework for generating pictographs that achieve appropriate fidelity between a visual representation and its corresponding concept. This framework is an evolution of Scott McCloud’s Big Triangle which began to suggest a method for semiotic precision.
Importantly, our research provides a method for refining this Trapezoid with potential users. The generative AI we envision utilizes an LLM, or large language model, to produce visual conceptual pegs. We determined verbal descriptors for axes of the trapezoid, and for vectors within it. This permits creation of Likert scales to validate the Pictorial Trapezoid’s positional scheme according to actual human beings’ interpretation of varied pictographs. And on the machine side, tight ranges of verbal description could be incorporated into training a model for restricting conceptual pegs to a range empirically determined to represent a sweet spot for human recall. (See article written for the Journal of Visible Language)
We also have a manuscript currently under review for the Design Research Society’s 2024 conference. This paper describes the deliberate process used in this project to organize and deconstruct the exploratory studies.
Finally, we are actively working on a white paper for LAS that considers future work. In this project we employed a pure design research process that was not prescriptive. Through our rigorous exploration we identified future research questions that are worth asking.
Please note: This material is based upon work done, in whole or in part, in coordination with the Department of Defense (DoD). Any opinions, findings, conclusions, or recommendations expressed in this material are those of the authors and do not necessarily reflect the views of the DoD and/or any agency or entity of the United States Government.
Text in this entry has been excerpted from “The Pictorial Trapezoid: Adapting McCloud’s Big Triangle for creative semiotic precision in generative text-to-image AI” authored by Matthew Peterson, Ashley Anderson, Kayla Rondinelli and Helen Armstrong
Scenario Video 1: Background Environment Concept
Persona: Cameron, a translator—an analyst who translates foreign language material into coherent English.
Scenario Video 2: Widget Concept
Persona: Sloane, a scanner—an analyst who identifies information or activity that answers all or part of an intelligence requirement and meets reporting thresholds.
Scenario Video 3: Sidecar Concept
Persona: Ferris, a QCer—an analyst who conducts quality control (QC) reviews of summaries and translations, and the reports that reference them.
Our Research Process:
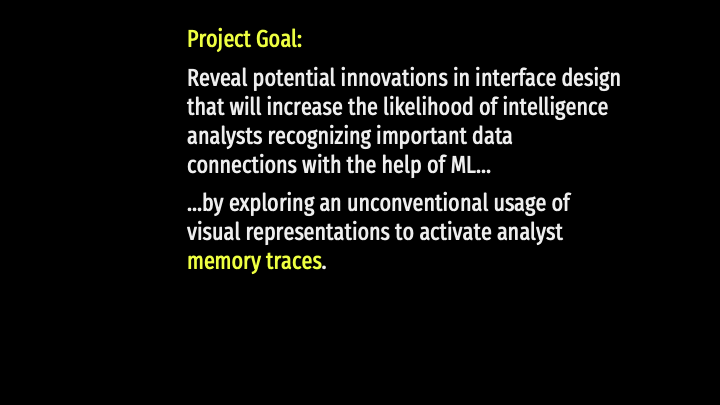
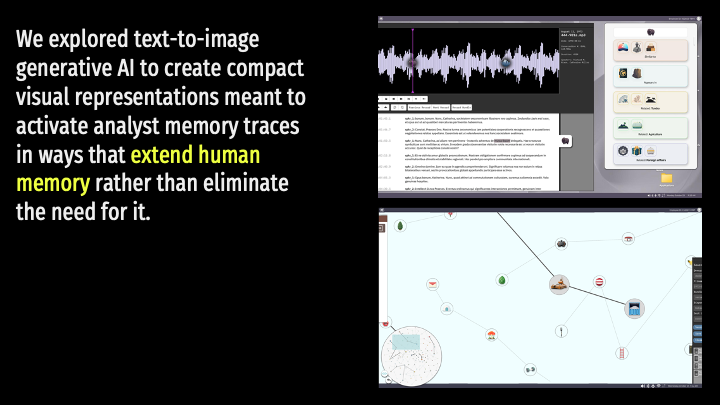

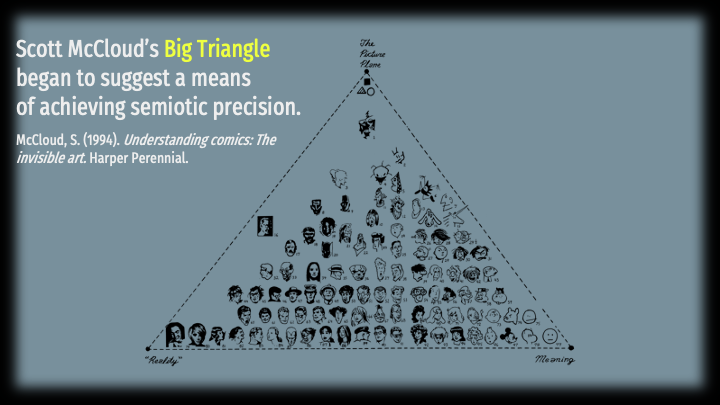
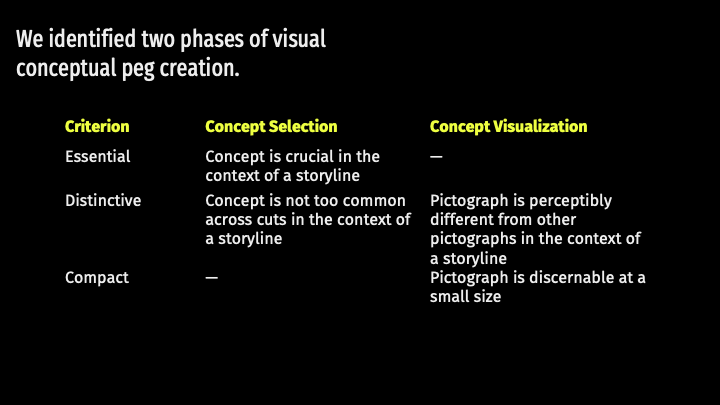
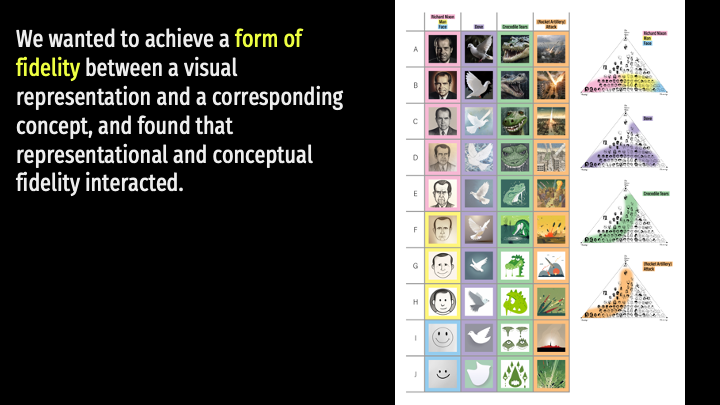
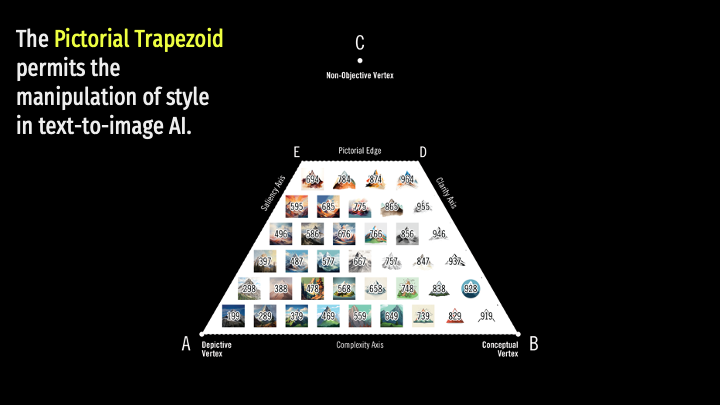
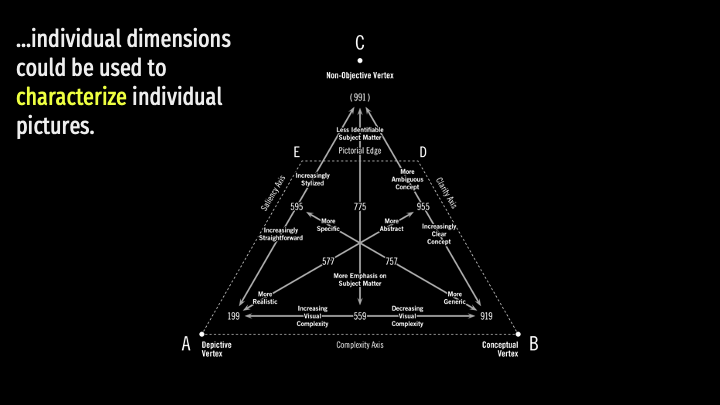
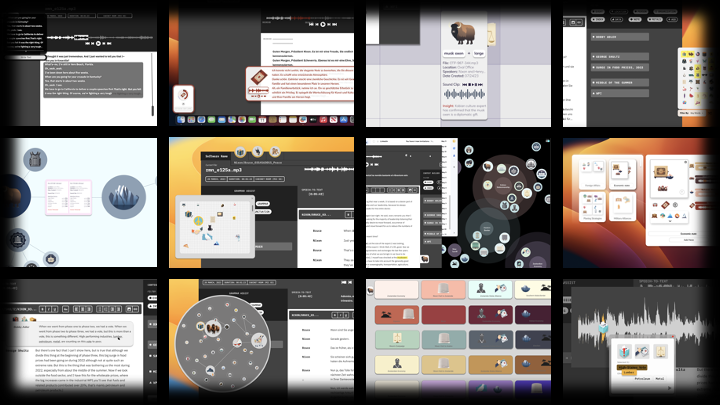

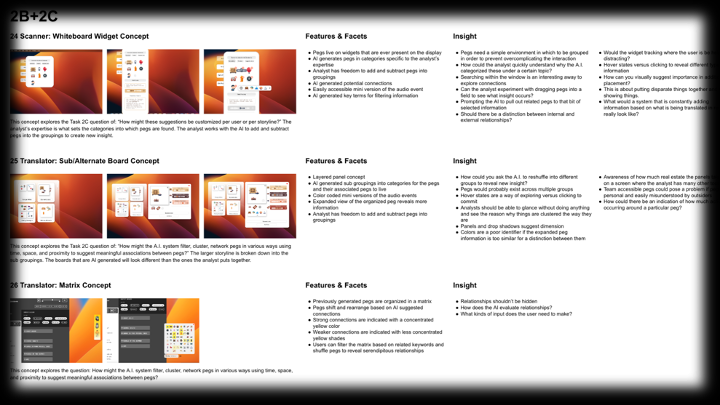
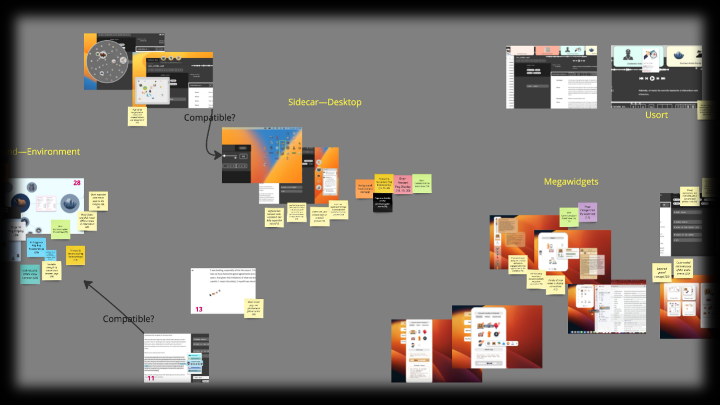
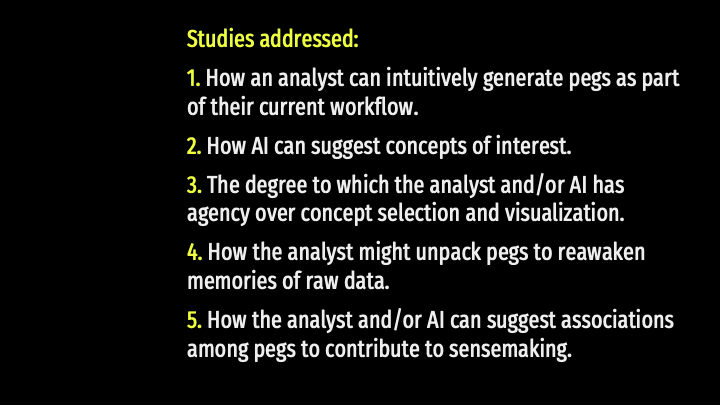
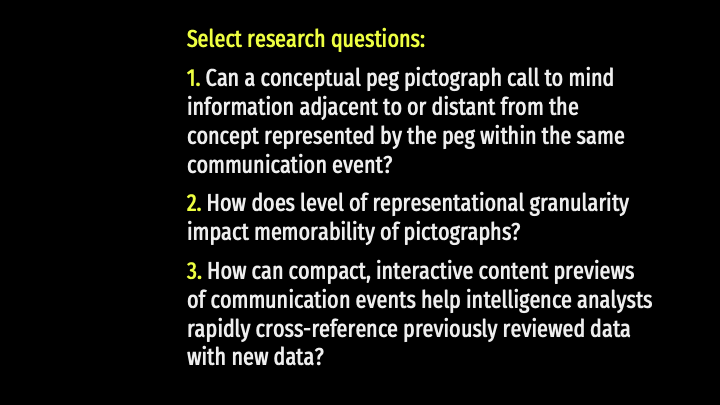